For On The Table 2015 I met with Heidi Massey and Ben Merriman over coffee and tea in the Loop. My idea for the conversation focused on creating an open consent form template — meaning, a web form users could finish and then export as a Memorandum of Understanding (MOU), a Non-Disclosure Agreement (NDA), or a Data Sharing Agreement (DSA).
The different documents work in different contexts. Except when working with datasets protected by federal law (more on this later), calling an agreement between parties an MOU or a DSA is largely a matter of habit, while an NDA is a legally binding contract that says which types of confidential information should not be disclosed. Within legal limits, there’s nothing stopping you from writing agreements for your organization in the language and structure you prefer. Consider the purpose of the dataset, who has stakes in its integrity, and what might happen to the dataset in the future.
Often boilerplate NDAs and MOUs are kept filed by organizations. An employee, consultant, or another partner adds their details to the agreement. Both parties sign the agreement and each keeps a copy for themselves. The agreement acts as a promise that, essentially, data stays where it belongs. Violations end the data sharing relationship.
We saw problems with agreements whose force relies on the color of law and a CYA — Cover Your Ass — mentality. So we tried to imagine how the language of the agreements could promote a culture of shared best practices. The conversation followed Heidi’s idea that small nonprofits have more in common with small businesses than they do with very large nonprofits. Here’s a plain English outline for a data agreement which also works like a data integrity check list.
People who are working with shared data should understand:
- How the data is formatted for use. This means organizing the dataset into simple tables and, for example, by using the same file type, naming conventions, and variable order.
- The versions of the dataset. An original version of the dataset should be kept unmodified. Changes to the dataset should be made to a copy of the original version and documented in detail. The location of the original version of the dataset should be known but access restricted.
- How long the data sharing agreement lasts. The dataset’s life cycle—how a dataset gets created, to where it can be transferred, and when, if at all, a dataset is destroyed–is just as important as a straightforward timeline for deliverables.
- How to keep information confidential. Avoiding accidental violations of the data sharing agreement is easier when everyone who works with the dataset is familiar with its terms of use. It’s possible to define access permissions to datasets by using password protection and defining read/write roles for users. Data cleaning is a crucial part of this process to ensure that personally identifiable information is kept safe.
- What costs come with sharing the data. This means being clear about who is in charge of updating the dataset, whether there are financial obligations associated with the data sharing process, and knowing risks associated with breaches. Federal law regulates the sharing of datasets about school children (FERPA), medical information (HIPPA), and vulnerable populations (IRBs).
- Specific use requirements. This is the nitty-gritty of data sharing. Use requirements specify whether a dataset can be shared with third parties, what other data (if any) can be linked to the dataset, and what changes can be made to the dataset.
Ben has written extensively about the consent process as it relates to the genetic material of vulnerable populations. A vulnerable person — say, a prisoner, child, or an indigenous person — consents to give a sample of their genetic material to a researcher for a study. The genetic material gets coded into a machine readable format and aggregated into a dataset with other samples. The researchers publish their study and offer the aggregated dataset to others for study.
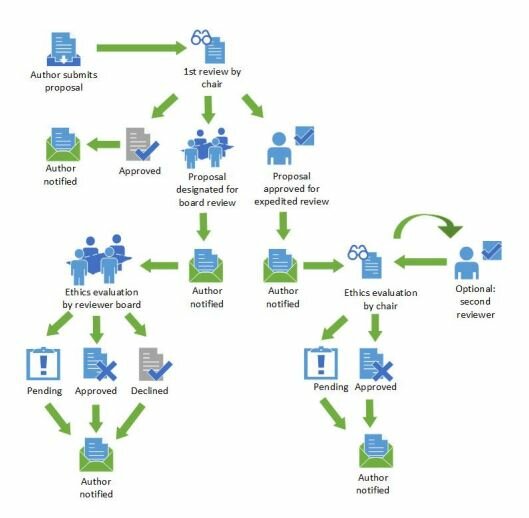
Image from Anne Bowser and Janice Tsai’s “Supporting Ethical Web Research: A New Research Ethics Review”. Copyright held by International World Wide Web Conference Committee: http://dx.doi.org/10.1145/2736277.2741654.
As it stands, though, there is no way for a person to revoke their consent once s/he gives away their genetic material. The dilemma applies not just to genetic material but any dataset that contains sensitive material. We thought people should have a say in what data counts as sensitive. An organization can limit how much data is shared in the first place. There are technical limitations and capacity limitations that stop people “in” datasets from having a voice during the dataset’s full life cycle.
For more information you can go to one of Smart Chicago’s meetups or review a list of informal groups here. The documentation is from last year’s Data Days conference as part of the Chicago School of Data project. There’s a large community in Chicago willing to teach people about data integrity. Check out Heidi’s resource list, which you can access and edit through Google.